Molecular typing prediction based on heterogeneous data
|
I. Main Contents
1.1 Brief Introduction
This project proposes a research project based on real clinical data, and analyzes multimodal brain MRI images and pathological images through fusion of deep learning techniques. WHO classification and molecular markers of glioma can be obtained before operation by exploring the imaging appearance of gene mutations so that the most reasonable diagnosis and treatment program was aided to formulate. By using the cross and fusion technology of image, pathology and multi-group information and establishing new theories, new approaches and new standards for intelligent diagnosis and precise treatment of tumors, this project can explore the technology and application mode of artificial intelligence aided diagnosis, precise treatment and prognosis judgment of tumors.
1.2 Purposes
1)Constructing a glioma molecular typing dataset containing multi-source heterogeneous information such as patient text information, MRI images and pathological images.
2)Observing the tumor lesions in the multimodal brain image to obtain the apparent information of the tumor.
3)Fusion of multi-source heterogeneous data for glioma WHO classification and glioma molecular marker prediction.
4)Developing a glioma-assisted diagnosis system, constructing a molecular marker-based glioma molecular typing knowledge base and inputing multi-source heterogeneous data to obtain the auxiliary diagnosis results of grading and molecular typing of glioma.
5)The clinical diagnostic evaluation will be carried out in the neurosurgical deployment of the Xiangya Hospital.
|
II. Research Plans
2.1 Dataset Establishment
Based on the historical medical data of Xiangya Hospital of Central South University. This project will select brain tumor related cases and test results to construct a multimodal glioma molecular typing dataset. The dataset is the world's first glioma molecular typing dataset based on multiple information including patient Text Data, MRI Images and Pathological Images. The number of cases to be constructed is more than 100 cases.
Sample of Glioma Molecular Typing Data Set as follows:
2.2 Recognition of Glioma Lesions based on Clinical Images
Clinical imaging examination of Glioma usually contains MRI images and Pathological images, which is an important means of early tumor diagnosis. Recognition of tumor lesions by imaging is an important basis for subsequent classification and molecular typing of tumors. However, the lesions in clinical images are usually blurred in boundaries and features are not obvious. Therefore, lesion identification is a task with high technical threshold and time consumption and there is subjective awareness risk in manual interpretation of images. Therefore, it is necessary to use intelligent algorithms to identify lesions and challenging work.
The structure of the deep learning network for segmentation designed in this project is shown in Figure 2. The full-supervised multi-modal three-dimensional full Convolutional Neural Network is used for feature extraction. Compared with the loss of resolution caused by the large number of sampling times in the traditional full Convolutional Neural Network. The whole hole convolution network expands the receptive field and reduces the number of downsampling by introducing a cavity convolution in the full convolutional network. More accurate segmentation results.
In addition, previous studies have shown that even excellent algorithms on open source medical MRI image datasets are difficult to migrate to actual clinical data. The reason is the development sequence, scanning resolution and machine magnetic field strength used in different images. The difference is caused by the difference between the two types of data and the algorithm is not universal. If the problem of mobility of the algorithm is not solved, the image-based intelligent auxiliary diagnosis can only stay in the laboratory. Therefore, this phase will focus on how to migrate algorithms based on open source image dataset training to less labeled or even unlabeled clinical data.
3D cavity convolution U-Net neural network structure is as follows:
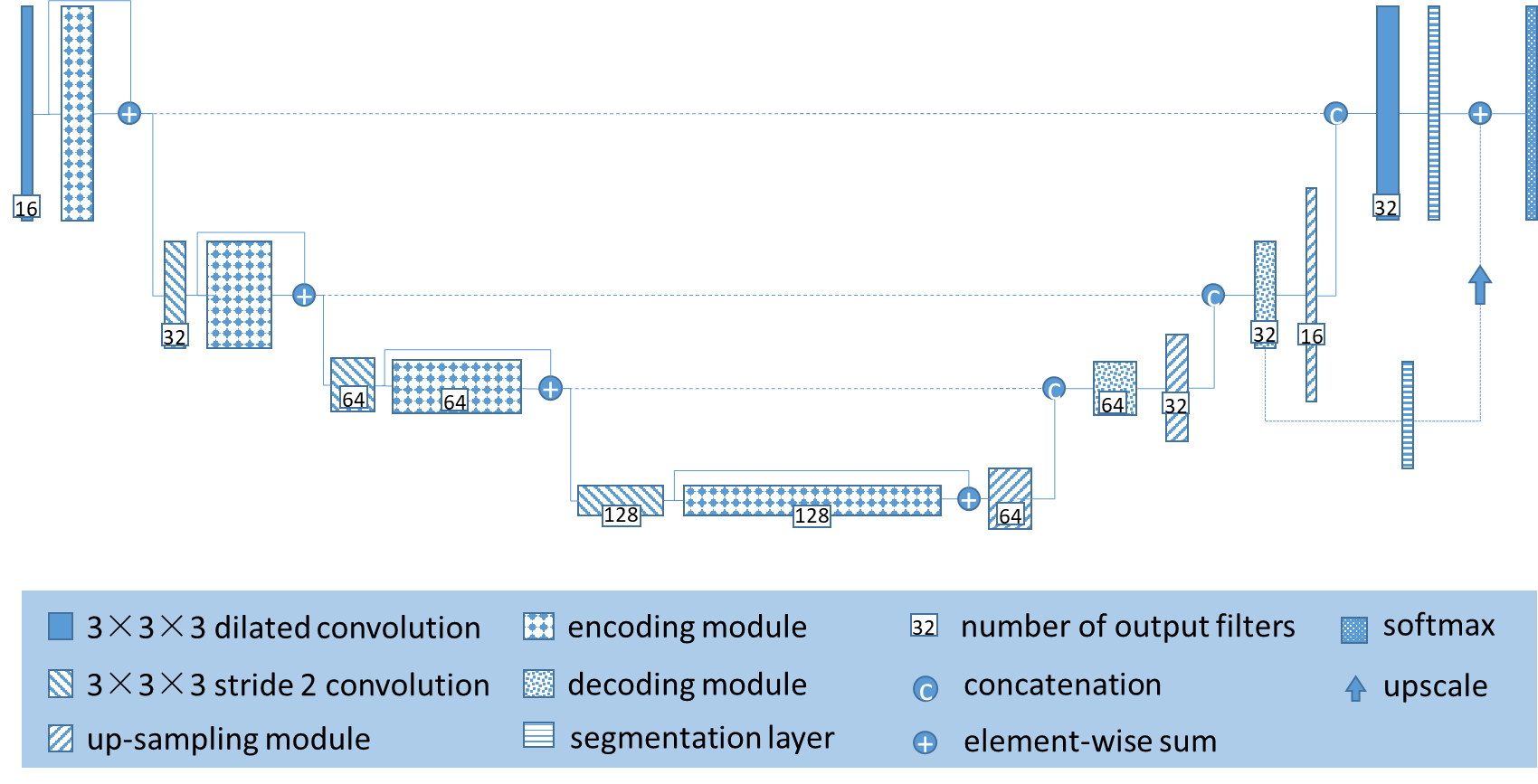
2.32.3 Fusion of Multi-Source Heterogeneous Information to predict Glioma Grading and Molecular Typing Results
Clinically, since no reliable relationship has been found between the molecular markers and the apparent features of the images, only the pathological examination of the excised tumor tissue can determine the tumor classification and molecular typing, in order to determine the classification before surgery and reduce the diagnostic cost. It is necessary to explore a feasible solution for predicting the classification of non-invasive information such as patient images and data; at the same time, some molecular mutations are difficult to detect even after pathology and need to be sequenced to determine the final time in order to reduce the time of detection and the cost of money. An algorithm for predicting molecular typing based on multi-source heterogeneous data added to pathological images also needs to be explored.
The structure of the integrated hybrid Deep Learning Network designed in this project is shown in Figure 3. The Residual Neural Network is designed based on the image. The output feature vector is integrated with the patient's additional information (such as age, gender, medical history, etc.) and the results of genetic testing and its prediction. In addition, the traditional methods are used to calculate the manual features, such as the gray value in the neighborhood, the mean within the neighborhood, the standard deviation and the low-frequency co-efficients of the two-dimensional wavelet transform. The original features and the image depth features are selected by Principal Component Analysis. The imaging features of each gene mutation are used as reference for future imaging diagnosis.
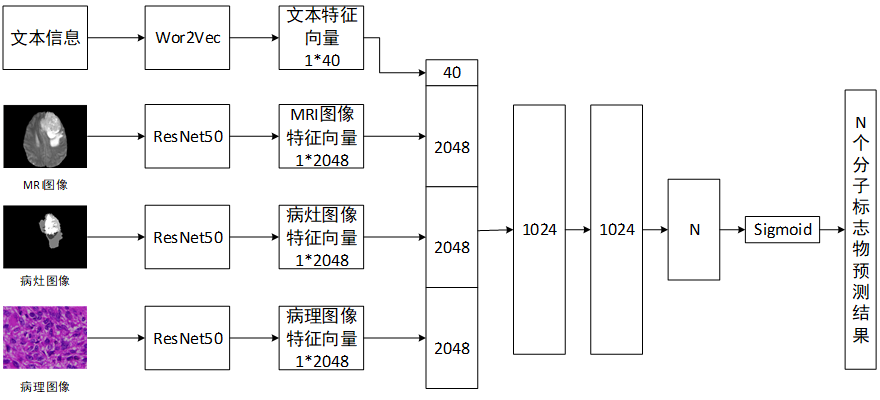
|
Copyright Notice
Please respect our original works. Please indicate the original source and author information of the article when reprinting. If you have any questions or ideas and would like to communicate with us, please use the email or phone at the bottom of the page to contact.
|
|