1. Database construction: We have established a five-year partnership with Beijing's at a glance NGO Center to collect a total of 4,444 scene images from 75 cities in 26 cities in China to build a database VAQI-1. This database is the largest scene image database for air quality detection that has been published so far.
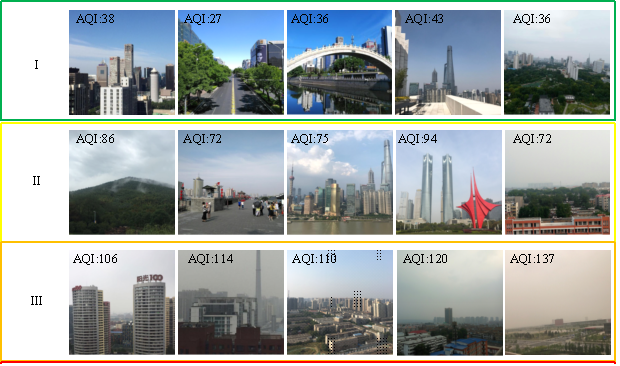
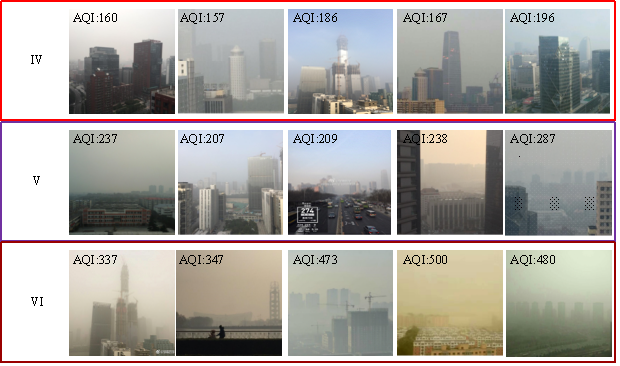
VAQI-1 Part Sample Picture
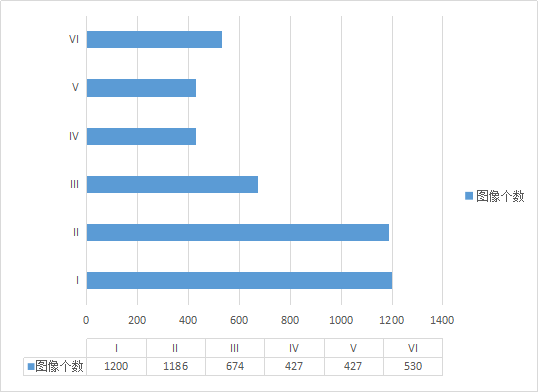
Number of Images of different Air Quality Levels in VVAQI-1
2. Estimating scene air quality based on a single image: Based on the basic assumptions of atmospheric physics, we can calculate the ideal atmospheric extinction coefficient for unpolluted air mass. By introducing image defogging technology to calculate the transmittance of arbitrary air quality and the non-polluting air mass transmittance of the scene and combining the obtained values of atmospheric extinction coefficient under the unpolluted air quality. The atmospheric extinction coefficient of any air quality in the scene can be obtained. Get the corresponding visibility value. The experiment proves that the method realizes the visibility of a single image by means of shooting information for the first time without complicated auxiliary information and has strong innovation. The results have been published in an EI conference paper applying for a patent for invention.
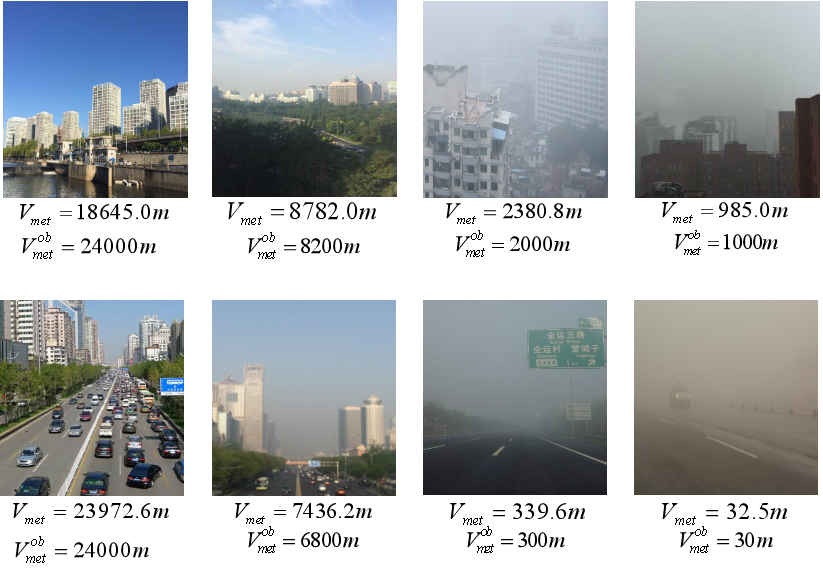
Visibility Estimation Result Example
3. Estimating Scene Air Quality Based on Image Feature Information: This project is based on the VAQI-1 database to extract image visual features (SIFT features, HOG features, LBP features, Color Saturation, Color Histograms and Contrast), Visibility Features and Convolution-Based Neural Networks. The depth features extracted by the network (LeNet model, AlexNet model, VGG model, GoogLeNet model and ResNet model) are studied by the multi-classification support vector machine method to study the relationship between these visual features and the scene air quality. The experimental results show that the accuracy of the air quality level estimation using the depth feature extracted by the fine-tuned GoogLeNet model reaches 76%. This result is not only superior to other air quality level estimation methods based on depth features but also superior to all air quality level estimation methods based on visual features and visibility features. This fully proves that this type of depth feature best fits the air quality image mechanism. The result has been applied for a patent for invention.
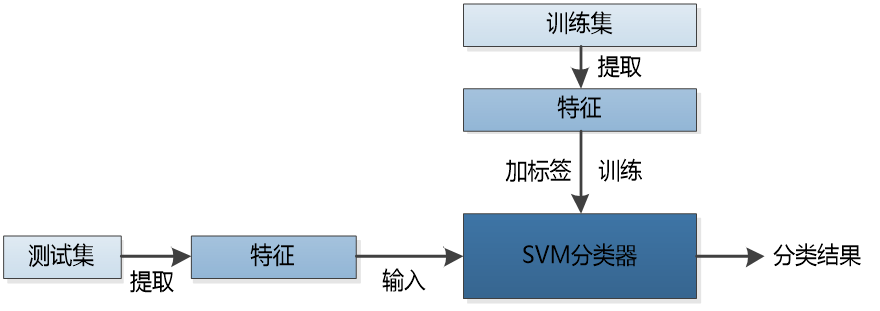
Air Quality Classification Framework Based on Image Features
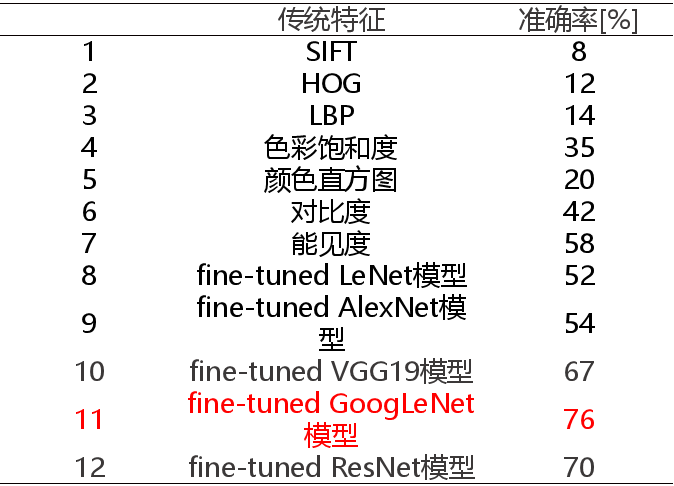
Comparison of Experimental Results of Different Image Features